Molecular Simulations & Machine Learning
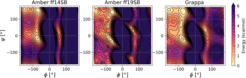
Image courtesy: Leif Seute, Eric Hartmann
To tackle the complexity of responsive biological or synthetic systems, we develop computational techniques tailored towards non-equilibrium and scale-bridging questions. These techniques encompass machine learning to handle chemical reactivity in classical Molecular Dynamics simulations or to improve force fields. Machine learning interatomic potentials for biomolecular and other soft matter systems is one of our goals. Atomistic simulations are our major work horse, and we employ coarse-graining or link to continuum methods wherever needed.
Scientists @ MPIP & collaborators involved
Friederich, Pascal, KIT
Projects
- SIMPLAIX, Klaus Tschira Foundation
- ERC Consolidator grant Radicol
- GRK 2450 Tailored Scale-Bridging Approaches to Computational Nanoscience