Alice Allen
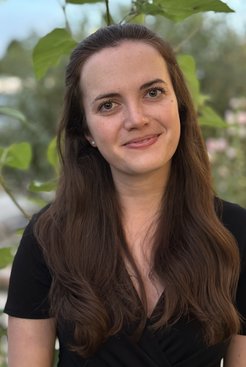
Dr. Alice Allen joined the Max Plank Institute of Polymer Research as a Group Leader at the beginning of 2025. She completed her undergraduate degree in Physics at Imperial College London and her PhD in Physics at the University of Cambridge. She was subsequently a research associate at the University of Cambridge and the University of Luxembourg before spending three years at Los Alamos Laboratory as a postdoctoral researcher. Her work has focused on developing new forms of molecular potentials, including machine learning potentials.
Research Interests
Machine learning models of interactions between atoms and molecules, known as interatomic potentials, are increasingly being used for atomistic simulations. A wide variety of machine learning models have been created to describe the interactions present in atomistic simulations. These models can be used to simulate reactive processes, shock physics and material properties. Our research group is dedicated to advancing machine learning methods and models to predict molecular properties and simulate chemical reactions accurately and efficiently. We develop models that can address a wide range of systems, including reactive processes and biological molecules.
Publications
Relevant Publications:
Allen, A. E. A.; Dusson, G.; Ortner, C.; Csányi, G.: Atomic permutationally invariant polynomials for fitting molecular force fields. Machine Learning: Science and Technology 2 (2), p. 025017 (2021)
Allen, A. E. A.; Tkatchenko, A.: Machine learning of material properties: Predictive and interpretable multilinear models. Science Advances 8 (18), p. eabm7185 (2022)
Matin, S.; Allen, A. E. A.; Smith, J.; Lubbers, N.; Jadrich, R. B.; Messerly, R.; Nebgen, B.; Li, Y. W.; Tretiak, S.; Barros, K.: Machine learning potentials with the iterative Boltzmann inversion: Training to experiment. Journal of Chemical Theory and Computation 20 (3), pp. 1274 - 1281 (2024)
Allen, A. E. A.; Csányi, G.: Toward transferable empirical valence bonds: Making classical force fields reactive. The Journal of Chemical Physics 160 (12), p. 124108 (2024)
Allen, A. E. A.; Lubbers, N.; Matin, S.; Smith, J.; Messerly, R.; Tretiak, S.; Barros, K.: Learning together: Towards foundation models for machine learning interatomic potentials with meta-learning. npj Computational Materials 10 (1), p. 154 (2024)
Recent Publications at MPIP:
No matching publications found.